CEE Ph.D. Defense Announcement: Machine Learning for the Prediction of PFAS Fate and Transport in the Natural and Built Environment
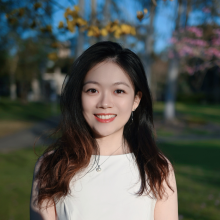
Jialin Dong, Ph.D. Candidate
UC Irvine, 2025
Assistant Professor Christopher Olivares Martinez
Abstract: Per- and polyfluoroalkyl substances (PFAS) are persistent synthetic chemicals widely used in industry and consumer products. Their persistence and mobility have led to widespread contamination of water and soil, posing health risks such as immune dysfunction and cancer. Complex environmental factors influence their spread, while traditional monitoring remains costly and inefficient. This research integrates big-data analytics with artificial intelligence (AI) to enhance PFAS monitoring, prediction and risk assessment. Machine learning (ML) is used to build predictive models that identify contamination patterns and estimate exposure risks, while large language models (LLMs) assist with data processing and knowledge synthesis. As one of the first efforts to apply AI to PFAS fate and transport analysis, this work advances scalable, data-driven strategies for long-term pollution control and public health protection.